AI Challenges and Risks: Ensuring Compliance & Safety
Discover AI challenges, risks, and compliance strategies in today's evolving landscape. Learn to balance innovation with regulation while ensuring AI safety.
share this
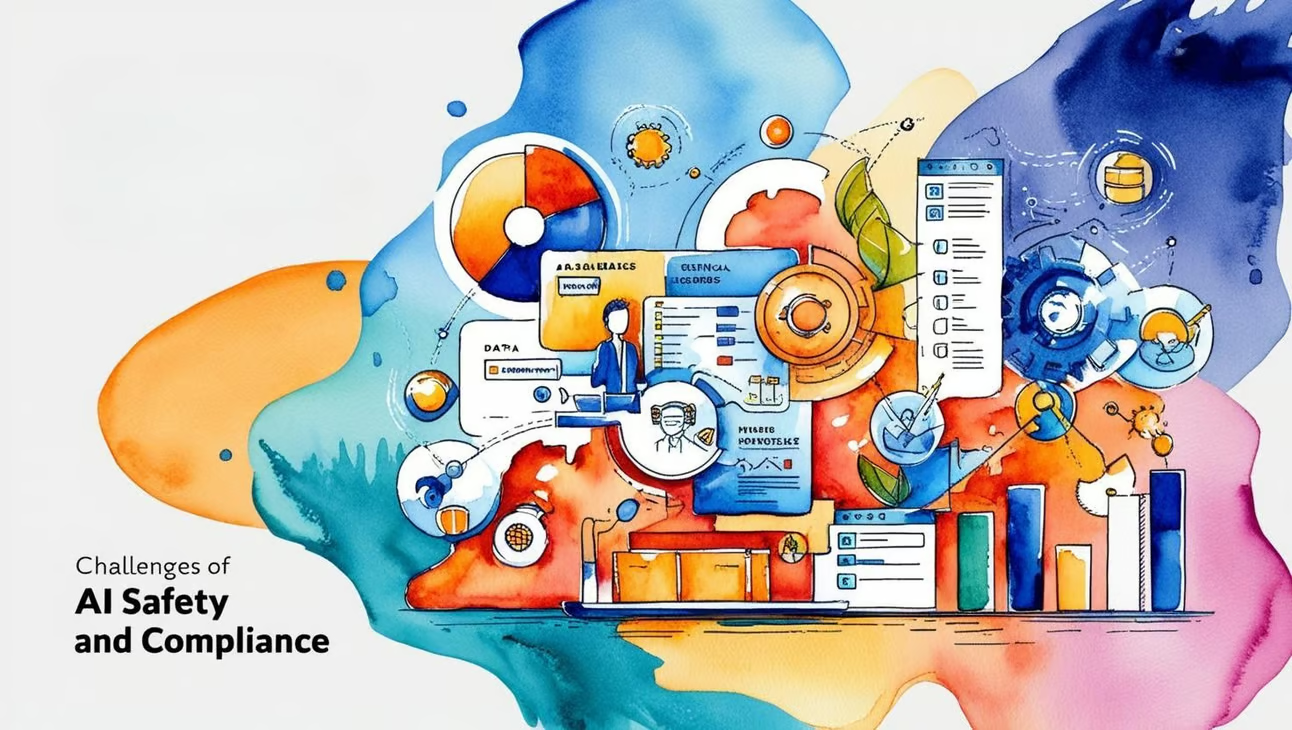
This newsletter is a summary of this podcast on ContinuousTV.
Host: Anand Natarajan, Director of AI/ML Solutions Delivery, xLM Continuous Intelligence
Guest: Justin Brochetti, CEO, Intelligence Factory
Justin Brochetti is the Co-Founder and CEO of Intelligence Factory, an applied AI company revolutionizing industries through advanced data retrieval, AI agents, and automation solutions. With over two decades of leadership experience in sales, marketing, and business development, Justin has built a reputation for innovation and integrity. Under his leadership, Intelligence Factory has developed groundbreaking AI solutions, such as Feeding Frenzy and Buffaly, that prioritize safety, compliance, and reliability in artificial intelligence applications.
1.0. Introduction: Challenges in AI Safety and Compliance
The rapid expansion of Artificial Intelligence (AI) presents both immense opportunities and significant risks. Despite its advancements, only 9% of organizations utilizing AI daily fully understand AI compliance and risk management. This "bias monitoring gap" results in AI bias, regulatory hurdles, and ethical dilemmas.
To mitigate these risks, frameworks like the European Union’s AI Act enforce AI safety and compliance standards. However, balancing innovation and regulation remains a challenge. A strategic approach—incorporating continuous AI monitoring, trust metrics, and bias detection—is crucial for ethical AI adoption. The cornerstone of effective risk management lies in continuous monitoring, as the principle "whatever is monitored is managed" serves as a guiding philosophy for AI compliance and security.
2.0. What Are the Biggest AI Failures?
AI failures can lead to financial losses, ethical concerns, and trust erosion. Here are some critical cases:
- IBM Watson’s Misdiagnosis: Resulted in a $62M loss due to AI miscalculations in healthcare.
- Zillow’s AI Pricing Model Flaw: A flawed AI valuation model led to an $881M financial loss.
- AI in Medical Imaging (COVID-19): Bias in training data resulted in incorrect diagnoses.
Even in non-critical sectors, AI errors in scheduling, moderation, and data analysis highlight the importance of AI governance and risk assessment.
3.0. The Regulatory Landscape: Ensuring AI Trust & Compliance
3.1. Why is AI Trust Important?
Trust in AI is defined by safety, transparency, and reliability. Leading tech firms use metrics such as:
- Microsoft's AI Trust Score (1-100 scale) to assess transparency.
- SHAP Values to explain AI decision-making processes.
- Compliance standards like GDPR and EU AI Act for ethical AI usage.
Industries like healthcare, finance, and autonomous vehicles demand stringent AI safety protocols, often requiring error margins below 1% to ensure trust.
3.2. Key AI Regulations to Watch
- European Union AI Act: Introduces risk-based AI classifications and compliance mandates.
- US NIST AI Risk Management Framework: Establishes safety protocols for AI systems.
- ISO AI Standards: Ensures global compliance with ethical AI practices.
4.0. Balancing AI Innovation with Regulation
While regulations prevent harm, overregulation may stifle AI advancements. To maintain equilibrium, companies should:
- Monitor AI models continuously using bias-detection tools.
- Implement ethical AI frameworks (e.g., fairness, accountability, transparency).
- Ensure explainability to build trust with stakeholders.
4.1. AI Safety Best Practices for Businesses
- Adopt a Risk-Based AI Strategy: Classify AI models based on risk levels.
- Regular Model Audits: Evaluate AI decision-making for biases.
- Enhance Explainability: Use SHAP and LIME for model transparency.
- Implement Continuous Monitoring: AI governance must be proactive, not reactive.
5. Conclusion
Successfully navigating the AI landscape requires a balanced approach that mitigates risks while leveraging opportunities. As AI technology evolves, so must our understanding of trust, transparency, and regulatory compliance. The future of responsible AI depends on our ability to harmonize safety with innovation, ensuring that AI-powered solutions are ethical, effective, and beneficial to society.
6.0. AI Latest News
- Three Types of Intelligence Explosion
- Y Combinator (YC), the renowned startup accelerator, is experiencing unprecedented growth and profitability among its current batch of startups, largely due to advancements in artificial intelligence (AI).
- Auditing Language Models for Hidden Objectives
7.0. FAQs
share this