AI-Powered Predictive Maintenance & Temperature Monitoring
Learn how Continuous Predictive Maintenance (cPdM) & Continuous Temperature Monitoring (cTM) enhance efficiency, reduce downtime, and ensure compliance.
share this

1.0. Introduction
With the rise of Industry 4.0, manufacturing and technology sectors are undergoing a groundbreaking transformation with the adoption of Continuous Predictive Maintenance (cPdM) and Continuous Temperature Monitoring (cTM). These innovative strategies mark a major shift from traditional maintenance and data management practices, leveraging an advanced AI tech stack to enhance operational efficiency and ensure regulatory compliance.
- By integrating artificial intelligence (AI) and machine learning (ML), organizations can optimize their processes, proactively address regulatory changes, and improve overall performance. Predictive maintenance solutions empower manufacturers to anticipate equipment failures, minimizing downtime and achieving cost savings of 30-50%.
- Simultaneously, cTM services are revolutionizing temperature mapping by automating data collection and analysis, ensuring compliance with stringent FDA regulations.
- Additionally, ContinuousGPT enhances workflow efficiency by enabling seamless interaction with data through conversational AI, facilitating faster decision-making and improved operational processes.
With these advancements, industry standards are being redefined. To address regulatory concerns, a human-in-the-loop approach is incorporated, ensuring compliance and reliability.
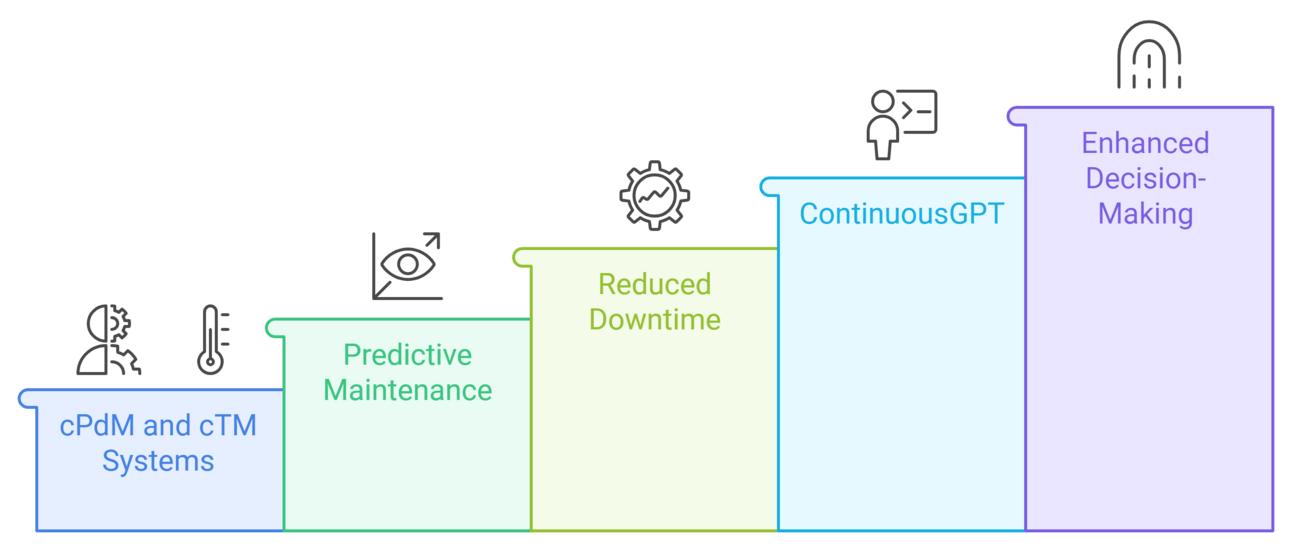
2.0. Continuous Predictive Maintenance (cPdM)
2.1. Introduction to cPdM
The manufacturing sector is undergoing a major shift in maintenance strategies, moving from traditional preventive maintenance (PM) to more advanced predictive maintenance (PdM) solutions. This transition is driven by the limitations of PM, which often leads to unnecessary downtime and suboptimal return on investment (ROI).
To address these challenges, manufacturers are embracing AI-driven predictive maintenance systems powered by machine learning (ML). By leveraging predictive analytics, companies can reduce maintenance costs by 30-50% through early failure detection, minimizing unexpected equipment breakdowns and maximizing asset efficiency.
At the forefront of this innovation is ContinuousPdM, a state-of-the-art platform designed for GxP compliance. This solution integrates real-time data sources with advanced analytics, offering tailored insights for engineers and operations teams to enable proactive decision-making and optimized maintenance schedules.
2.1.1. Key Steps in Implementing Predictive Maintenance
Implementing predictive maintenance (PdM) requires a well-structured approach, including:
- Cross-functional team formation for PdM execution.
- Comprehensive asset assessments to identify critical equipment.
- IoT sensor deployment to monitor real-time performance metrics.
- AI-powered predictive models for failure detection.
- Centralized monitoring systems for real-time equipment tracking.
By utilizing advanced ML techniques, such as time-series forecasting and anomaly detection, manufacturers can significantly improve their failure prediction accuracy and enhance operational efficiency.
2.1.2 Benefits of Continuous Predictive Maintenance (cPdM)
- Minimized Unplanned Downtime – Detect failures before they occur.
- Optimized Asset Performance – Ensure smooth and efficient operations.
- Cost Reduction – Lower maintenance costs and extend asset lifespan.
- Regulatory Compliance – Meets GxP and Industry 4.0 standards.
AI and ML play a crucial role in Industry 4.0, revolutionizing smart manufacturing with real-time predictive maintenance. Companies leveraging cPdM benefit from data-driven insights, enhanced efficiency, and sustainable profitability.
2.2. PdM Workflow
Developing an effective predictive maintenance model involves several critical steps to improve maintenance scheduling, minimize downtime, and enhance operational efficiency.

2.2.1. Data Gathering:
Effective predictive maintenance (PdM) relies on data collection to anticipate equipment failures. The primary sources include:
- Sensor Data – Vibration, sound, and temperature readings from accelerometers, ultrasonic microphones, and built-in process sensors help detect early signs of failure.
- CMMS (Computerized Maintenance Management Systems) Data – Historical maintenance logs, part replacements, and downtime trends provide insights into failure patterns and help estimate Remaining Useful Life (RUL).
By combining real-time sensor inputs with historical maintenance data, businesses can enhance asset reliability, reduce downtime, and optimize maintenance strategies.
2.2.2. Data Pre-processing:
To ensure accurate predictive maintenance, raw data must be cleaned and structured before being used in machine learning models. Key steps include:
- Handling Missing Data – Methods like deletion, mean/median imputation, and statistical estimation techniques (e.g., Maximum Likelihood Estimation) help fill data gaps.
- Outlier Detection – Statistical models and anomaly detection techniques (e.g., Local Outlier Factor, Isolation Forest) identify irregularities while preserving critical failure indicators.
- Normalization – Standardization techniques like z-score normalization, log transformation, and min-max scaling improve data consistency and enhance model performance.
Proper data preprocessing ensures higher accuracy in failure predictions, optimizing maintenance strategies and minimizing unexpected downtime.
2.2.3. Feature Engineering:
Feature engineering plays a vital role in enhancing predictive maintenance models by converting raw data into actionable insights. Key techniques include:
- Time-Based Features – Methods like lag analysis, time series decomposition, and stationarity tests help capture trends and temporal patterns.
- Frequency-Based Features – Autocorrelation and spectral analysis identify cyclic behaviors and repeating patterns in sensor data.
- Feature Engineering for Classification – Techniques such as Principal Component Analysis (PCA) and t-Distributed Stochastic Neighbor Embedding (t-SNE) simplify data while retaining essential information, improving model accuracy.
Effective feature engineering enhances failure prediction accuracy, leading to proactive maintenance and reduced downtime.
2.2.4. Machine Learning (ML) Modeling:
ML models form the backbone of predictive maintenance, enabling accurate failure predictions. Key steps include:
- Time Series Forecasting – Models like ARIMA and Long Short-Term Memory (LSTM) networks predict future sensor values by detecting trends and seasonal patterns.
- Error Classification – Algorithms such as Support Vector Machines (SVMs), Decision Trees, and Random Forests classify equipment conditions and failure types.
- Anomaly Detection – Methods like Isolation Forest and Local Outlier Factor (LOF) identify irregular patterns signaling potential issues.
Implementing these ML techniques enhances maintenance efficiency, reducing unexpected failures and operational disruptions.
2.2.5. Dashboarding and Reporting:
Interactive dashboards provide real-time insights, improving decision-making across teams:
- Engineering Dashboard – Analyzes tool wear, torque, and temperature to detect failure modes and optimize maintenance costs.
- Operations Dashboard – Helps technicians predict failures, reduce downtime, and sustain production efficiency.
- Facilities Management Dashboard – Tracks KPIs like Mean Time Between Failures (MTBF), Mean Time to Repair (MTTR), and maintenance costs for better resource planning.
Visualizing data simplifies complex insights, making predictive maintenance more effective and actionable.
Key Reports for PdM
- Zone Report: Summarizes asset performance metrics for engineers.

- Sensor Report: Analyzes specific sensor data within operational zones.
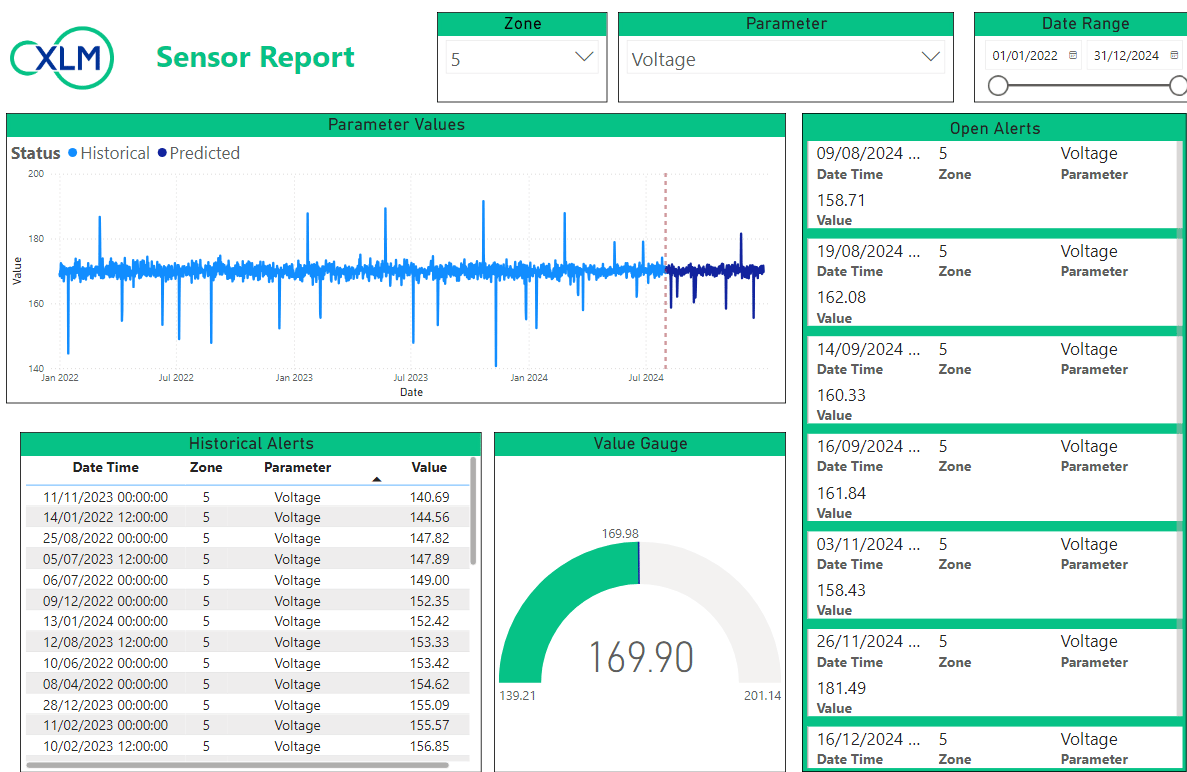
- Insights Overview Report: Tracks project status through issue identification and resolution KPIs.
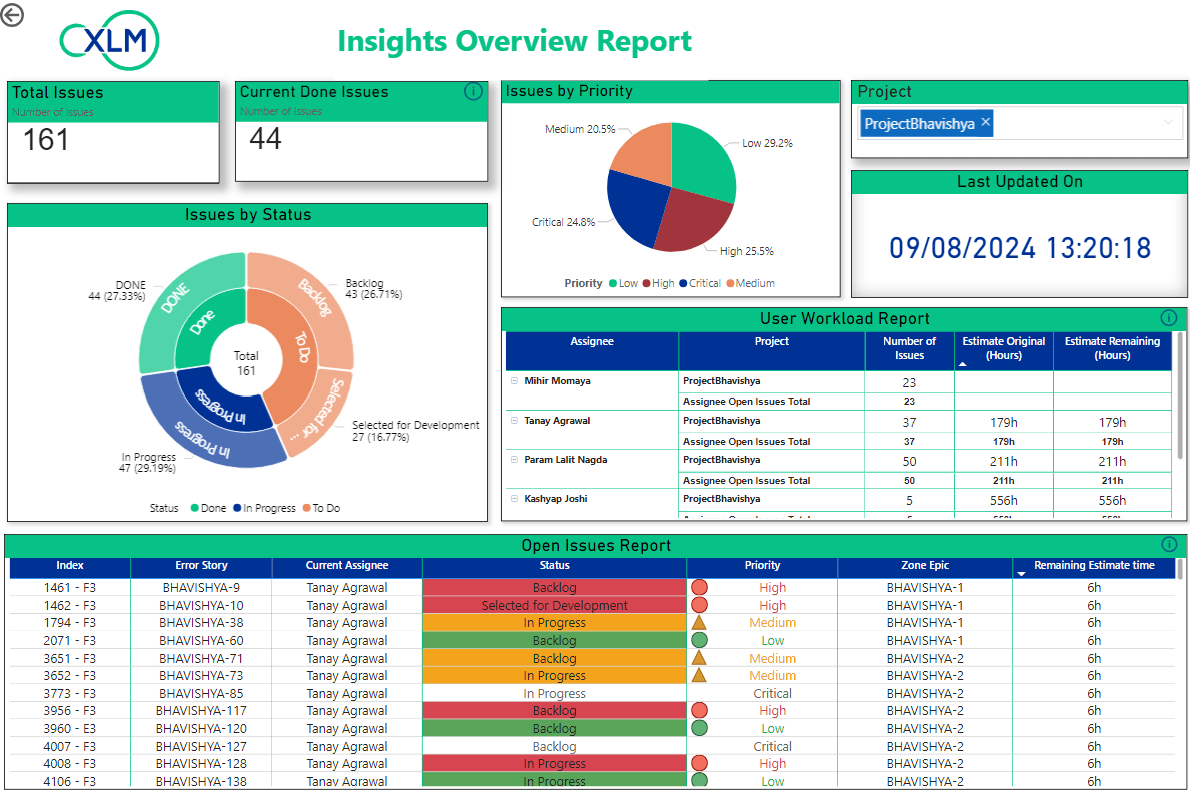
2.3. Use of Large Language Models (LLMs) in PdM
Large Language Models (LLMs) are transforming predictive maintenance by enabling advanced data analysis, automation, and real-time insights. Their key applications include:
- Chatbots for Maintenance Support – Assist engineers with context-aware responses by analyzing equipment logs and manuals.
- Automated KPI Reporting – Generate real-time reports on machine performance with visual insights.
- Dynamic Data Exploration – Enable users to interactively analyze trends and failure patterns through natural language queries.
By integrating LLMs, organizations can streamline maintenance workflows, enhance operational efficiency, and make data-driven decisions faster.
2.4. Why Choose cPdM?
Continuous Predictive Maintenance (cPdM) offers numerous advantages that optimize industrial operations and enhance efficiency. Key benefits include:
- Cost Reduction – Lowers maintenance expenses by preventing unexpected failures.
- Improved Decision-Making – Provides data-driven insights for proactive strategies.
- Minimized Downtime – Ensures proactive maintenance scheduling to avoid disruptions.
- Enhanced Asset Performance – Extends equipment lifespan through optimized maintenance.
- Integration with Advanced Technologies – Enables seamless tool integration for enhanced efficiency.
- Automation of Workflows – Reduces manual errors, improving reliability.
- Enhanced Visualization Tools – Simplifies complex data interpretation for better insights.
- Streamlined Communication and Collaboration – Supports a centralized ticketing system for better coordination.
- Comprehensive Analytics and Reporting – Offers robust performance tracking for continuous improvement.
By leveraging cPdM, businesses can achieve predictive efficiency, reduce costs, and drive smarter maintenance strategies aligned with Industry 4.0.

3.0. Continuous Temperature Monitoring (cTM)
3.1. Introduction to cTM
Continuous Temperature Monitoring (cTM) is an advanced solution designed to enhance temperature monitoring in regulated environments. Industries handling temperature-sensitive products face stringent compliance requirements, making precise environmental control essential.
cTM automates data collection and analysis, ensuring compliance with regulatory standards while improving accuracy and operational efficiency. The system features three key dashboards:
- Fixed Sensors Dashboard – Tracks data from permanently installed sensors, highlighting temperature trends and key performance indicators (KPIs).
- Temporary Sensors Dashboard – Uses calibrated NFC or RF data loggers to monitor environmental conditions and generate compliance reports.
- Sensor Mapping Dashboard – Compares data from fixed and temporary sensors, providing a comprehensive overview of storage conditions.
By replacing manual monitoring methods, cTM improves data reliability, enhances compliance, and streamlines operations. Its predictive analytics capabilities help identify temperature fluctuations early, ensuring optimal storage conditions and regulatory adherence.
3.1.1. Understanding the cTM Dashboards:
cTM includes two primary dashboards—the Temporary Sensors Dashboard and the Fixed Sensors Dashboard—each designed for specific sensor types. Together, they provide a complete view of storage conditions.
- Temporary Sensors Dashboard: This dashboard captures data from calibrated NFC or RF data loggers to monitor temperature and humidity. Key features include:
- Recorded Extremes – Displays highest and lowest temperatures along with specific locations.
- Detailed Data Tables – Organizes readings by date, time, logger ID, and temperature.
- Daily Summaries – Highlights trends for quick analysis.
- Timeline Visualizations – Tracks temperature fluctuations over time.
- Deviation Graphs – Assesses sensor accuracy.
- Comparative Analysis – Uses T-tests to ensure data reliability.
- Recorded Extremes – Displays highest and lowest temperatures along with specific locations.
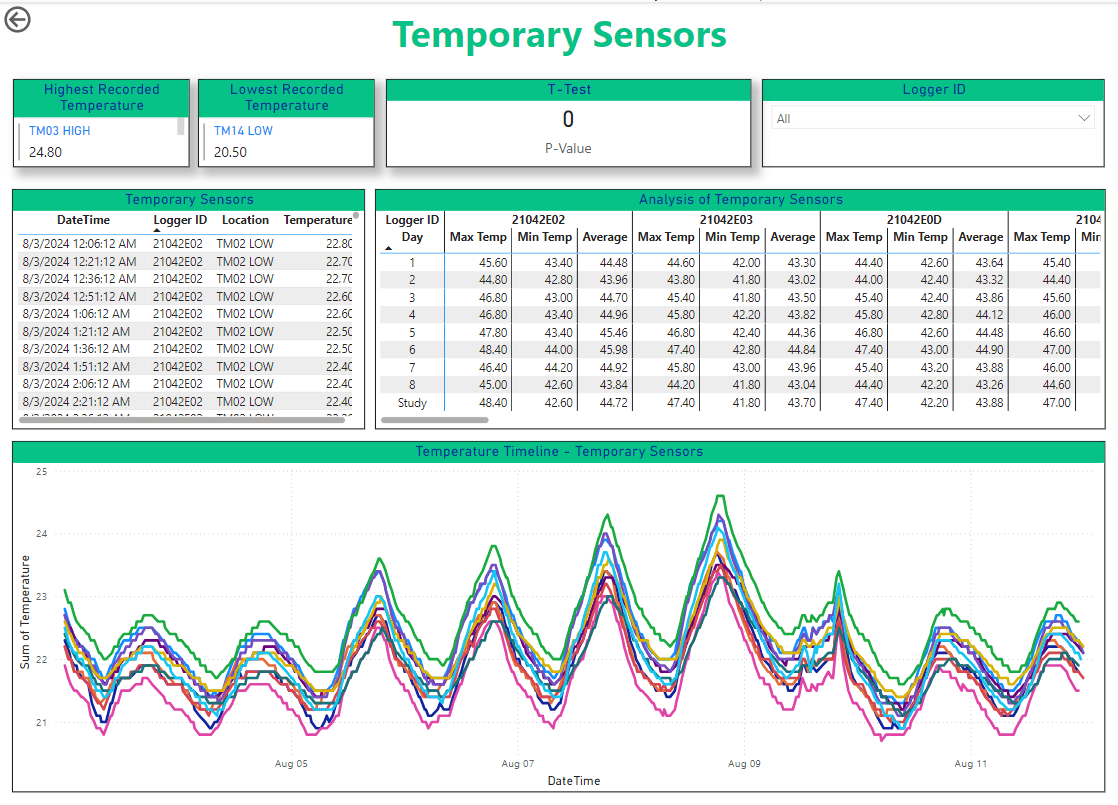
- Fixed Sensors Dashboard: This dashboard focuses on data from permanently installed sensors, offering similar functionality as the Temporary Sensors Dashboard but tailored for fixed monitoring requirements.

- Sensor Mapping Dashboard: Designed for comparing data from both fixed and temporary sensors, this dashboard uses proximity-based grouping to enhance interpretation. It includes:
- Temperature Comparison Graphs – Provides side-by-side analysis of sensor readings.
- Deviation Analysis – Identifies inconsistencies for improved accuracy.
- Automated Data Processing – Streamlines insights and enhances reliability.
- Temperature Comparison Graphs – Provides side-by-side analysis of sensor readings.
By integrating these dashboards, cTM ensures precise monitoring and compliance with regulatory standards while improving operational efficiency.
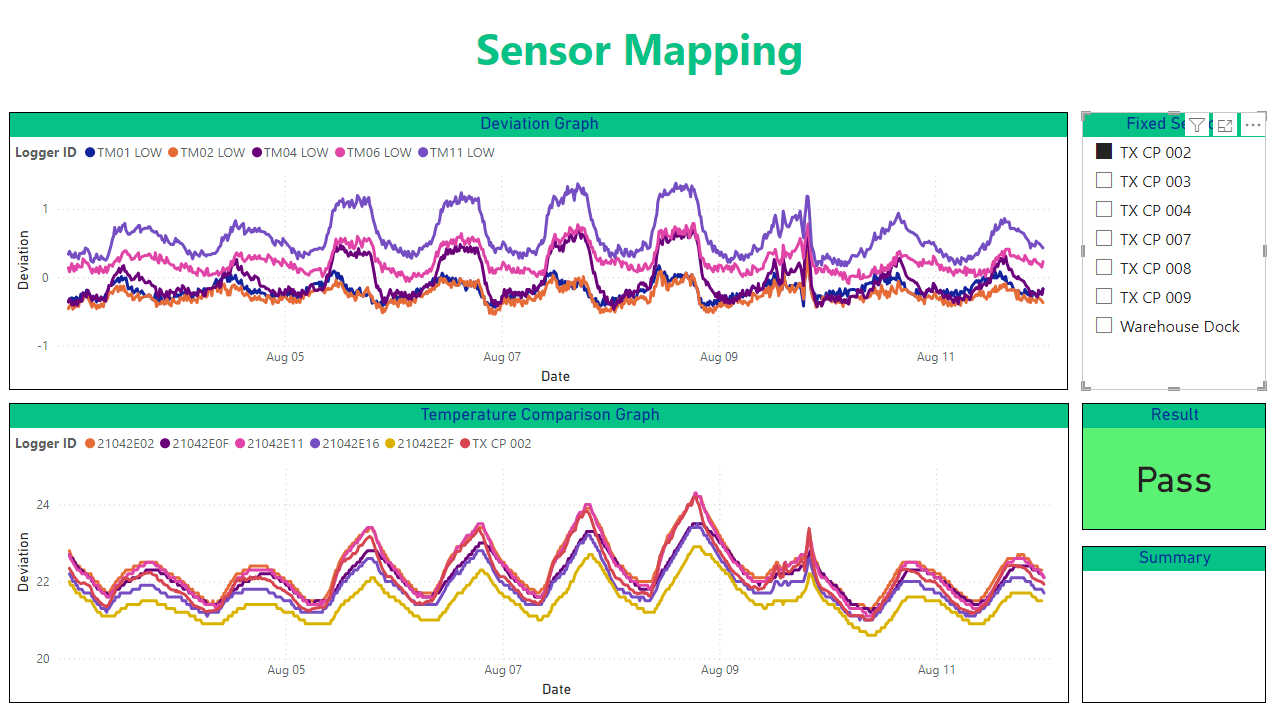
3.1.2. The Power of Data Automation and ML:
cTM enhances temperature mapping accuracy and ensures regulatory compliance through advanced data automation and ML techniques. Key processes include:
- Data Labeling and Transformation – Converts raw sensor data into standardized, structured datasets, improving consistency and usability.
- Data Pre-processing – Applies normalization techniques and feature engineering to refine data for accurate forecasting and analysis.
- ML-Driven Automation – Uses predictive analytics for temperature trends, anomaly detection for sensor malfunctions, and automated reporting for real-time insights.
By integrating these capabilities, cTM streamlines compliance, enhances operational efficiency, and reduces manual intervention.
3.2. Why cTM?
Continuous Temperature Mapping (cTM) offers numerous advantages, making it a vital solution for industries requiring precise environmental monitoring.
3.2.1. Key Benefits:
- Enhanced Compliance – Ensures adherence to regulatory standards and mitigates risk.
- Improved Accuracy – Provides real-time precision monitoring for temperature-sensitive environments.
- Predictive Analytics – Enables proactive management and anomaly detection, reducing the likelihood of system failures.
- Operational Efficiency – Automates data collection, minimizing human error and improving workflow.
- Streamlined Data Management – Standardizes datasets and enables automated data processing for better decision-making.
- Detailed Trend Analysis – Identifies patterns and trends through visualized temperature data.
- Reliability and Validation – Enhances data validation with comparative analysis for increased trustworthiness.
- Comprehensive Data Insights – Leverages advanced dashboards for real-time monitoring and reporting.
- Cost-Effectiveness – Reduces labor and operational costs while optimizing resource utilization.
By integrating automation and advanced analytics, cTM enhances reliability, compliance, and efficiency, making it an essential tool for controlled environments.
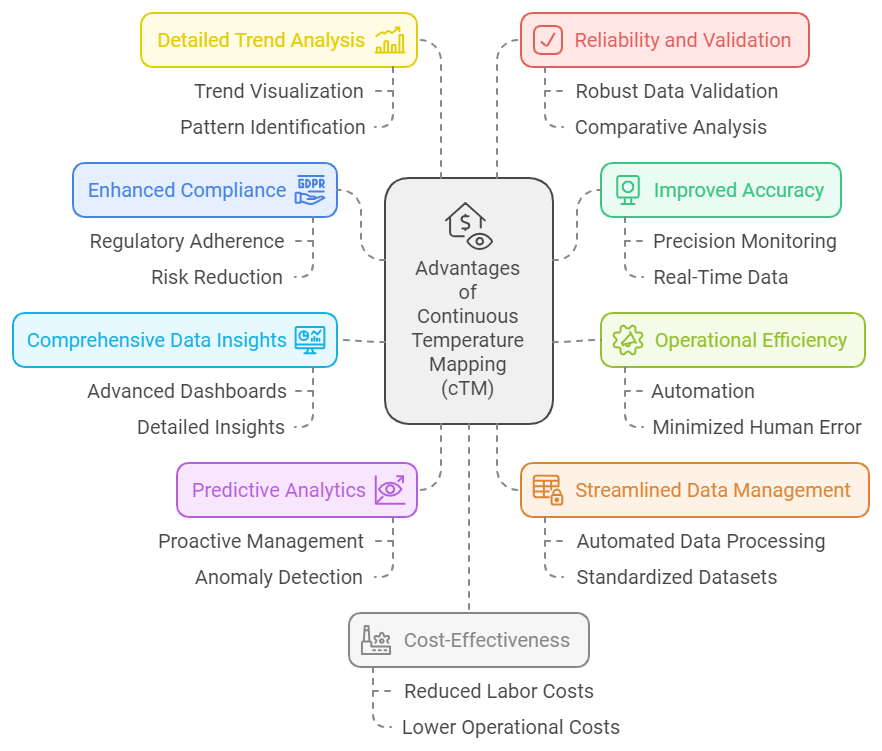
4.0. ContinuousGPT (cGPT)
4.1. Introduction to cGPT
ContinuousGPT (cGPT) is transforming workflows in the life sciences industry by enabling conversational data interaction, enhancing efficiency, and improving decision-making.
4.1.1. Key Features of cGPT:
- Conversational Data Interaction – Users can engage with data through natural language queries, simplifying access to complex insights.
- Retrieval-Augmented Generation (RAG) – Enhances data retrieval accuracy by integrating real-time and contextual information.
- Knowledge Graphs – Strengthens information structuring, enabling intelligent data linking and improving user experience.
- Security and Compliance – Ensures data privacy, regulatory adherence, and controlled access within highly regulated environments.
By combining AI-driven automation with advanced retrieval techniques, cGPT optimizes data accessibility and workflow efficiency, making it a powerful tool for modern life sciences applications.
4.2. Why cGPT?
ContinuousGPT (cGPT) revolutionizes data retrieval and interaction in life sciences by offering a secure, efficient, and AI-powered approach to managing large datasets. The key benefits include:
1. Efficient Querying
- AI-driven natural language processing (NLP) enables fast and accurate search results.
- Eliminates manual effort in navigating complex databases.
2. Enhanced Data Accessibility
- Enables seamless access to critical data across repositories.
- Ensures real-time information retrieval for better decision-making.
3. Seamless Communication
- Facilitates collaborative interactions with AI agents tailored to enterprise needs.
- Supports conversational queries for intuitive engagement.
4. Improved Workflow Efficiency
- Reduces time spent on data retrieval, optimizing productivity.
- Enhances regulatory compliance by streamlining access to validated information.
5. Streamlined Data Management
- Automates data organization and processing, minimizing redundancy.
- Supports structured knowledge representation for better insights.
6. Advanced Conversational Interactions
- AI-powered chatbots offer dynamic responses tailored to specific domains.
- Ensures context-aware interactions with retained chat history.
7. Secure Access and Compliance
- Azure AD authentication ensures role-based access and data security.
- Complies with regulatory standards in life sciences.
8. Integration with Popular Platforms
- Seamlessly connects with OneDrive, Teams, Confluence, and Veeva Vault.
- Provides a unified interface for multi-platform data retrieval.
9. Retrieval-Augmented Generation (RAG) Technology
- Enhances AI responses by combining LLMs with real-time data retrieval.
- Ensures accuracy, relevance, and contextual intelligence.
ContinuousGPT bridges the gap between data and decision-making, empowering life science enterprises with faster insights, smarter workflows, and enhanced compliance.

5.0. Conclusion
The shift towards Continuous Predictive Maintenance (cPdM) and Continuous Temperature Monitoring (cTM) is transforming industrial and regulated environments. These data-driven maintenance solutions enhance efficiency, reduce downtime, and improve equipment reliability by shifting from reactive to proactive strategies.
By leveraging predictive maintenance technology, businesses can optimize asset performance, minimize failures, and significantly cut operational costs. Real-time monitoring and data-driven insights allow organizations to improve compliance, streamline workflows, and enhance decision-making.
Furthermore, ContinuousGPT (cGPT) is revolutionizing data accessibility and workflow automation. With seamless integration across platforms, secure access, and conversational data interactions, cGPT enhances productivity and ensures users can efficiently retrieve critical information when needed.
Industry 4.0 is driving digital transformation in smart manufacturing. Businesses leveraging predictive maintenance, continuous monitoring, and AI-driven data insights will secure a competitive edge and long-term success. Organizations investing in these innovations will gain a competitive advantage by improving operational efficiency, ensuring compliance, and optimizing performance.
6.0. ContinuousTV Audio Podcasts
- AP003: What is cDI? The NextGen IT Stack for Life Sciences
- AP004: Can your ITOM do this?
- AP005: Can your SDLC do this?
7.0. Latest AI News
- 𝗔𝗿𝗲 𝘆𝗼𝘂 𝗿𝗲𝗮𝗱𝘆 𝗳𝗼𝗿 𝘁𝗵𝗲 𝗵𝗮𝗿𝘀𝗵 𝗿𝗲𝗮𝗹𝗶𝘁𝘆 𝗼𝗳 𝗔𝗜 𝘀𝗲𝗰𝘂𝗿𝗶𝘁𝘆 𝗶𝗻 𝟮𝟬𝟮𝟰? 𝗧𝗵𝗲 𝟮𝟬𝟮𝟰 𝗦𝘁𝗮𝘁𝗲 𝗼𝗳 𝗔𝗜 𝗦𝗲𝗰𝘂𝗿𝗶𝘁𝘆 𝗥𝗲𝗽𝗼𝗿𝘁
- 𝗚𝗲𝗻𝗲𝗿𝗮𝘁𝗶𝘃𝗲 𝗮𝗿𝘁𝗶𝗳𝗶𝗰𝗶𝗮𝗹 𝗶𝗻𝘁𝗲𝗹𝗹𝗶𝗴𝗲𝗻𝗰𝗲 𝗽𝗲𝗿𝗳𝗼𝗿𝗺𝘀 𝗿𝘂𝗱𝗶𝗺𝗲𝗻𝘁𝗮𝗿𝘆 𝘀𝘁𝗿𝘂𝗰𝘁𝘂𝗿𝗮𝗹 𝗯𝗶𝗼𝗹𝗼𝗴𝘆 𝗺𝗼𝗱𝗲𝗹𝗶𝗻𝗴
- 𝗔𝗿𝗲 𝗰𝘂𝗿𝗿𝗲𝗻𝘁 𝗹𝗮𝗻𝗴𝘂𝗮𝗴𝗲 𝗺𝗼𝗱𝗲𝗹𝘀 𝗿𝗲𝗮𝗱𝘆 𝘁𝗼 𝗱𝗶𝗮𝗴𝗻𝗼𝘀𝗲 𝗮𝗻𝗱 𝘁𝗿𝗲𝗮𝘁 𝗽𝗮𝘁𝗶𝗲𝗻𝘁𝘀? 𝗜𝗻𝘁𝗿𝗼𝗱𝘂𝗰𝗶𝗻𝗴 𝗠𝗘𝗗𝗜𝗖: 𝗮 𝗿𝗲𝘃𝗼𝗹𝘂𝘁𝗶𝗼𝗻𝗮𝗿𝘆 𝗳𝗿𝗮𝗺𝗲𝘄𝗼𝗿𝗸 𝘁𝗼 𝗳𝗶𝗻𝗱 𝗼𝘂𝘁!
8.0. FAQs
share this