Industrial DataOps: Real-Time Insights for Industry 4.0
Unlock real-time insights with Industrial DataOps. Boost efficiency, ensure compliance, and drive smart manufacturing with data-driven decisions in Industry 4.0
share this

1.0. Introduction to Industrial DataOps: Enhancing Data Management in Manufacturing
Industrial DataOps is a transformative methodology designed to improve the speed, usability, and reliability of data in manufacturing environments. By effectively managing the vast amounts of data generated from sensors, machinery, and control systems, Industrial DataOps empowers organizations to make faster, data-driven decisions and enhance operational efficiency.

1.1. What is Industrial DataOps?
Industrial DataOps refers to the systematic integration of people, processes, and technology to create a unified data infrastructure. This framework streamlines data workflows, enabling real-time decision-making and fostering continuous improvement.
- People: Promotes collaboration between IT, data engineers, and operations teams.
- Processes: Implements standardized data pipelines to ensure consistency and accuracy.
- Technology: Leverages advanced analytics, automation, and machine learning for actionable insights.
By orchestrating these elements, Industrial DataOps transforms raw, fragmented data into meaningful insights that drive operational efficiency and business innovation.
1.2. Best Practices for Solving Data Complexity in Industrial DataOps
Modern manufacturing generates massive and complex data sets, including:
- Sensor Data: Vibration, temperature, and flow rate readings.
- Machinery Logs: Performance metrics and maintenance history.
- Control Systems: Data from SCADA, PLCs, and MES platforms.
Industrial DataOps addresses the challenge of integrating and interpreting diverse data sources by:
- Centralizing Data Streams: Combines data from multiple systems into a unified platform.
- Ensuring Data Consistency: Applies standardized models for seamless interoperability.
- Reducing Silos: Enhances cross-team collaboration with shared, real-time insights.
This structured approach improves data quality and accessibility, enabling manufacturers to identify inefficiencies, optimize production, and reduce downtime.
1.3. How DataOps is Powering Industry 4.0 Transformation
As manufacturing evolves toward Industry 4.0, Industrial DataOps plays a pivotal role in:
- Enabling Smart Factories: Real-time data insights optimize production processes and enhance automation.
- Enhancing Predictive Maintenance: Continuous data monitoring reduces equipment failures and extends asset lifespan.
- Boosting Agility: Real-time analytics empower faster, data-driven decision-making.
- Ensuring Data Security and Compliance: Robust governance frameworks protect sensitive industrial data.
By embracing Industrial DataOps, organizations can achieve greater scalability, operational efficiency, and resilience in the face of rapid technological advancements.
2.0. Key Components of Industrial DataOps
The core components of Industrial DataOps form the foundation for efficient, reliable, and scalable data management in modern manufacturing environments. These elements ensure that industrial data is effectively processed, contextualized, and analyzed to drive informed decision-making.
2.1. Data Standardization: The Key to Seamless Industry Integration
Standardized data models are essential for consistent data interpretation and seamless integration across various manufacturing systems and devices.
- Uniform Data Models: Ensures compatibility between diverse equipment protocols (e.g., PLCs, SCADA, and ERP systems).
- Interoperability Across Platforms: Standardization facilitates smooth data exchange, reducing silos.
- Enhanced Data Consistency: Improves data quality and reliability for downstream analytics.
By implementing data standardization, organizations can streamline data processing and achieve better accuracy in predictive maintenance and quality control.
2.2. Contextualization for Enhanced Data Relevance
Contextualizing data ensures its relevance and usability for specific applications by analyzing it in relation to its source and intended use.
- Metadata Enrichment: Adds contextual tags (e.g., machine ID, timestamp, location) to raw data.
- Enhanced Traceability: Improves data lineage tracking and operational insights.
- Application-Specific Insights: Increases the accuracy of predictive analytics models by providing relevant context.
This data contextualization enables manufacturers to derive meaningful insights and make informed, real-time decisions.
2.3. Real-Time Analytics for Agile Operations
Real-time analytics is a cornerstone of Industrial DataOps, enabling manufacturers to rapidly adapt to market demands and operational challenges.
- Instant Data Processing: Reduces latency by analyzing data streams in real-time.
- Faster Decision-Making: Enhances agility by identifying issues early.
- Automated Anomaly Detection: Flags deviations immediately, minimizing downtime.
- Agile Methodologies: Improves the flexibility and speed of data handling processes.
With real-time analytics, organizations can proactively respond to inefficiencies, boosting overall equipment effectiveness (OEE) and productivity.
3.0. Benefits of Industrial DataOps
Implementing an Industrial DataOps framework offers a wide range of benefits that significantly enhance manufacturing efficiency, collaboration, and data security. Here’s how organizations can leverage it for better outcomes:
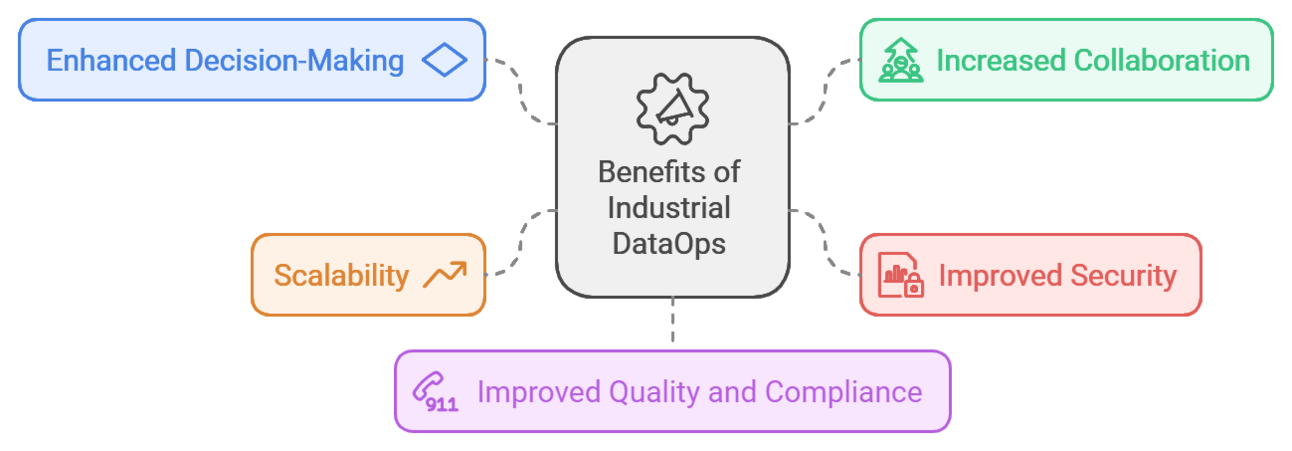
3.1. Enhanced Decision-Making with Real-Time Insights
By providing quicker access to refined data analytics, Industrial DataOps empowers organizations to make informed, data-driven decisions that optimize production processes and minimize downtime.
- Real-Time Analytics: Enables immediate responses to operational inefficiencies.
- Faster Root Cause Analysis: Identifies and resolves issues before they escalate.
- Improved Production Efficiency: Reduces bottlenecks through data-backed decision-making.
3.2. Increased Collaboration Across Teams
Industrial DataOps fosters a collaborative data ecosystem, breaking down silos and promoting seamless communication among diverse stakeholders, including:
- Engineers: Gain access to real-time machine performance data.
- Data Scientists: Utilize clean, contextualized data for accurate predictive models.
- Business Analysts: Leverage insights for strategic decision-making.
This collaborative atmosphere enhances productivity by ensuring all teams have access to consistent and reliable data.
3.3. Improved Data Security and Governance
Recognizing the sensitivity of industrial data, Industrial DataOps integrates robust security protocols to safeguard against cyber threats and unauthorized access.
- Role-Based Access Control (RBAC): Ensures only authorized personnel access sensitive data.
- End-to-End Encryption: Protects data during transfer and storage.
- Data Integrity Monitoring: Detects and prevents data tampering.
These advanced security measures ensure compliance with industry regulations such as FDA, GDPR, and ISO 27001.
3.4. Scalability for Growing Operations
As manufacturing operations expand, Industrial DataOps offers unmatched scalability, enabling organizations to:
- Seamlessly integrate new machines, sensors, and processes.
- Expand data pipelines without compromising performance.
- Adapt to changing market demands without major infrastructure overhauls.
This flexibility makes Industrial DataOps ideal for scaling production capabilities while maintaining operational efficiency.
3.5. Improved Data Quality and Compliance
Industrial DataOps adopts a "quality-by-design" strategy that ensures data accuracy and consistency throughout workflows.
- Automated Data Cleansing: Reduces human error by identifying and correcting inconsistencies.
- Continuous Pipeline Monitoring: Enhances observability and identifies quality issues swiftly.
- Automated Governance and Audit Trails: Ensures compliance with FDA, GDPR, and other regulatory standards.
- Comprehensive Documentation: Facilitates streamlined audits with clear data lineage and usage reports.
This approach improves data reliability, ensuring accurate reporting and minimizing compliance risks.
4.0. Industrial DataOps Use Case: Transforming Pharmaceutical Manufacturing
In the pharmaceutical manufacturing industry, Industrial DataOps is driving significant improvements in efficiency, compliance, and innovation. Here’s a real-world case study showcasing how a leading pharmaceutical company successfully implemented Industrial DataOps to overcome operational challenges and boost performance.
4.1. Case Study Overview
4.1.1. Company Background:
A top-tier pharmaceutical company faced mounting issues with:
- Data silos that hindered cross-department collaboration.
- Inefficient data management practices slowing down production insights.
- Compliance complexities with Good Manufacturing Practices (GMP) and other industry regulations.
The company aimed to harness its data more effectively to streamline operations, enhance product quality, and ensure compliance.
4.1.2. Implementation of Industrial DataOps:
1. Data Integration and Standardization
- The company deployed an Industrial DataOps platform with a centralized data abstraction layer.
- This layer enabled seamless integration of data from PLCs, sensors, and LIMS (Laboratory Information Management Systems).
- Standardized data models ensured uniform data representation, simplifying analysis and improving accessibility.
2. Real-Time Analytics for Enhanced Efficiency
- The company implemented real-time analytics to monitor key performance indicators (KPIs) such as:
- Yield rates
- Batch cycle times
- Equipment utilization
- By using advanced analytics tools, operators quickly identified inefficiencies and deviations from expected metrics.
3. Strengthened Compliance and Governance
- With the highly regulated pharmaceutical sector in mind, the company embedded robust data governance into the platform.
- The system ensured compliance with GMP regulations through:
- Centralized policy enforcement across manufacturing sites.
- Automated data lineage tracking and audit trails.
- This streamlined audit readiness and reduced the risk of regulatory violations.
4. Fostering Collaboration Across Teams
- Breaking down data silos improved information sharing between production, quality assurance, and R&D teams.
- This collaborative environment accelerated innovation and streamlined process optimizations.
5. Predictive Maintenance for Reduced Downtime
- The company implemented predictive maintenance strategies using real-time and historical sensor data.
- By detecting potential equipment failures early, they:
- Minimized unplanned downtime.
- Reduced maintenance costs through proactive servicing.
6. Scalable and Flexible Framework
- As the company expanded its operations and introduced new product lines, the Industrial DataOps framework scaled effortlessly.
- The platform enabled quick integration of new machines and processes without disruptions.
- This flexibility supported the company’s growth and adaptability to market demands.
4.2. Results Achieved with Industrial DataOps
The implementation of Industrial DataOps in the pharmaceutical company yielded several tangible benefits, significantly enhancing efficiency, compliance, and cost-effectiveness.

4.2.1. Key Measurable Improvements:
1. Reduced Time-to-Market:
- By leveraging real-time insights from production data, the company accelerated its product development cycles.
- Faster data analysis enabled quicker decision-making, reducing delays and boosting productivity.
2. Increased Operational Efficiency:
- The company improved Overall Equipment Effectiveness (OEE) by 15%.
- Real-time monitoring and data-driven optimizations helped streamline manufacturing processes and minimize downtime.
3. Enhanced Compliance and Audit Readiness:
- With automated data governance, the company reduced audit preparation time by 30%.
- Improved data lineage tracking and consistent policy enforcement ensured seamless regulatory compliance.
4. Significant Cost Savings:
- Through predictive maintenance, the company lowered maintenance costs by approximately 20%.
- Early fault detection and proactive servicing reduced unplanned downtime and expensive repairs.
4.2.2. Key Takeaway:
The Industrial DataOps framework delivered substantial business value, enabling the company to:
- Accelerate product launches with faster development cycles.
- Boost operational efficiency with real-time insights.
- Strengthen compliance with streamlined audit processes.
- Reduce costs through predictive maintenance strategies.
This case study highlights how Industrial DataOps is a game-changer in pharmaceutical manufacturing, driving smarter operations and ensuring consistent regulatory compliance.
5.0. Conclusion: Why Industrial DataOps is the Future of Manufacturing
In today’s data-centric industrial landscape, implementing Industrial DataOps is no longer a luxury—it’s a strategic necessity. By focusing on data standardization, contextualization, and real-time analytics, manufacturers can efficiently manage complex data ecosystems and gain a competitive edge.
5.1. Key Benefits of Industrial DataOps
Smarter, Faster Decision-Making:
- With real-time access to refined analytics, organizations can make quicker, data-driven decisions.
- This boosts production efficiency and minimizes downtime.
Stronger Collaboration Across Teams:
- DataOps frameworks break down data silos, enabling seamless cross-team collaboration.
- Engineers, data scientists, and business analysts can easily share insights and drive innovation together.
Enhanced Security and Compliance:
- Robust security protocols protect sensitive industrial data from cyber threats.
- Automated governance ensures regulatory compliance with standards like FDA, GMP, and GDPR.
Scalability and Flexibility:
- Scalable DataOps solutions allow manufacturers to easily integrate new machines, processes, and technologies.
- This adaptability ensures that operations stay agile and future-ready.
Continuous Improvement:
- Real-time monitoring and feedback loops enable organizations to refine strategies based on actual performance data.
- This iterative approach enhances predictive maintenance and fosters a culture of continuous innovation.
5.2. The Future of Manufacturing with Industrial DataOps
As the industry accelerates its shift towards Industry 4.0, the role of Industrial DataOps will only become more vital. Organizations that embrace this framework will be better positioned to:
- Leverage their data to optimize operations and reduce costs.
- Ensure regulatory compliance with automated governance.
- Gain a competitive edge through innovation and efficiency.
By prioritizing automation, collaboration, and real-time insights, manufacturers can unlock the full potential of their industrial data, driving long-term success in an increasingly data-driven world.
6.0. Related Publications
- #015: Can your PM do this? - Part 1
- #016: Can your PM do this? - Part II
- #025: Can your PM really do this - Part III?
7.0. ContinuousTV Audio Podcasts
- AP005: Can your SDLC do this?
- AP006: Can your AI/ML Stack do this? Predict, Monitor and Retrieve.
- AP007: Is your PLC an MTP based on Margo?
8.0. Latest AI News
- 𝗠𝗜𝗧 𝗠𝗲𝗱𝗶𝗮 𝗟𝗮𝗯: 𝗘𝘃𝗲𝗿 𝘄𝗼𝗻𝗱𝗲𝗿𝗲𝗱 𝘄𝗵𝗮𝘁 𝗮𝗱𝘃𝗶𝗰𝗲 𝗮 𝟲𝟬-𝘆𝗲𝗮𝗿-𝗼𝗹𝗱 𝘆𝗼𝘂 𝘄𝗼𝘂𝗹𝗱 𝗴𝗶𝘃𝗲 𝘁𝗼 𝘆𝗼𝘂𝗿 𝗽𝗿𝗲𝘀𝗲𝗻𝘁 𝘀𝗲𝗹𝗳? 🌟
- 𝗜𝗺𝗮𝗴𝗶𝗻𝗲 𝘁𝗿𝗮𝗻𝘀𝗳𝗼𝗿𝗺𝗶𝗻𝗴 𝗮 𝘀𝗶𝗻𝗴𝗹𝗲 𝗶𝗺𝗮𝗴𝗲 𝗶𝗻𝘁𝗼 𝗮 𝘀𝘁𝘂𝗻𝗻𝗶𝗻𝗴, 𝗿𝗲𝗮𝗹𝗶𝘀𝘁𝗶𝗰 𝘃𝗶𝗱𝗲𝗼 𝘄𝗶𝘁𝗵 𝗷𝘂𝘀𝘁 𝗮 𝗳𝗲𝘄 𝗰𝗹𝗶𝗰𝗸𝘀
9.0. FAQs
share this