Why Hybrid AI is the Future of Intelligent Systems and AI
Discover how Hybrid AI, combining machine learning and operations research, is transforming intelligent systems and solving complex optimization challenges.
share this
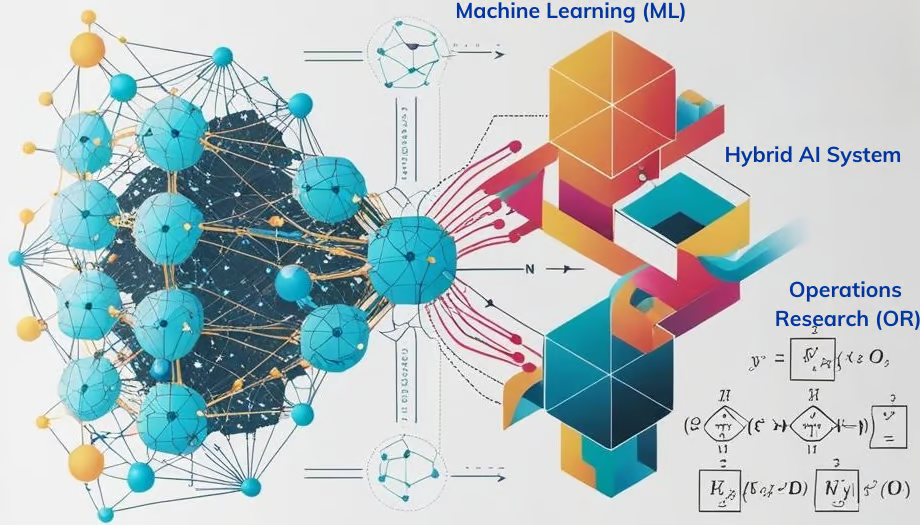
This newsletter is a summary of this podcast.
Host: Anand Natarajan, Director of AI/ML Solutions Delivery, xLM Continuous Intelligence
Guest: Dr. Nikolaj Van Omme, Founder & CEO, Funartech
Dr. Nikolaj van Omme is the chief executive officer of Funartech, an artificial intelligence startup that provides creative solutions to (very) complex industrial problems by combining machine learning and operations research (applied mathematics). He is a strong proponent of hybrid methods and approaches, in particular the hybridization of machine learning and operations research, for which he is a global pioneer.
1.0. Introduction: Why Hybrid AI is the Future of Optimization
In the fast-evolving world of artificial intelligence (AI), one question remains a hot topic: can machine learning (ML) tackle every optimization problem? The answer is no. Despite its success, machine learning (ML) has limitations, especially in addressing complex, real-world challenges. Enter hybrid AI, an innovative fusion of machine learning (ML), operations research (OR), and advanced mathematical methodologies. This integration is set to transform the future of artificial intelligence.
2.0. The Power of Operations Research in Hybrid AI
Operations research (OR) has been a crucial tool for optimization since its inception during World War II, helping to solve logistical and resource allocation problems. Despite its importance, OR is often overshadowed by newer technologies like ML. However, many ML techniques—particularly optimization algorithms—have their roots in OR.
In industries like aviation, OR is indispensable. Airlines use it for efficient flight path planning, crew scheduling, and aircraft maintenance. In contrast to ML, OR offers more reliable optimization for these complex problems.
3.0. Why Machine Learning Alone Falls Short in Optimization
Machine learning is a powerful tool for recognizing patterns and predicting trends. However, it has several significant drawbacks when applied to optimization:
- Data Dependency: ML requires vast amounts of quality data, which many industries lack.
- Lack of Robustness: ML models can produce inconsistent results when dealing with noisy or incomplete data.
- Black Box Nature: The algorithms used in ML are notoriously difficult to interpret, complicating the explanation of their decision-making processes.
- Limited Optimization Capabilities: While ML typically relies on brute-force search methods, operations research (OR) can achieve global optimization with significantly greater efficiency.
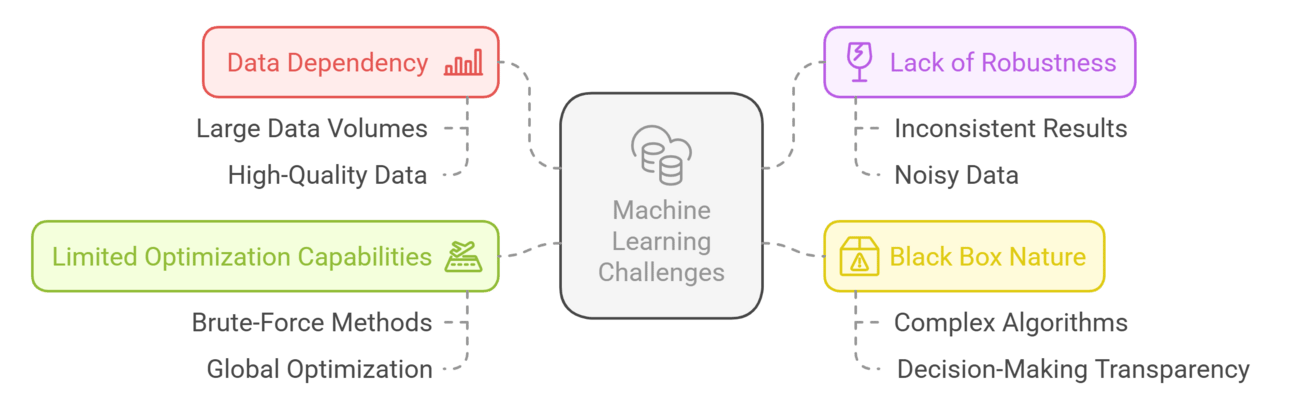
Exclusively depending on ML for critical applications, such as healthcare or supply chain management, carries substantial risks—ensuring accuracy and robustness is essential.
4.0. The Hybrid AI Revolution: A Game-Changer in Optimization
Hybrid AI signifies a transformative shift by harnessing the strengths of various disciplines. By merging the data-driven insights of machine learning (ML) with the mathematical precision of operations research (OR), hybrid AI systems can deliver significantly more reliable and efficient solutions.
For instance, Toyota faced an issue with optimizing the transportation of automotive parts across a global supply chain. While traditional ML methods had failed for two years, the integration of ML and OR brought a breakthrough. By using a cost-effective, small-model hybrid AI approach, Toyota reduced transportation costs by 30%, and optimized the supply chain in just minutes on a standard laptop.
5.0. Case Study: Toyota's Global Supply Chain Optimization Using Hybrid AI
Toyota's global supply chain faced the challenge of coordinating automotive components across continents. Traditional ML methods struggled to balance costs, inventory levels, and delivery schedules. However, a hybrid AI solution combining ML and OR proved highly effective.
- ML analyzed historical and real-time data to predict demand trends and optimize transportation routes.
- OR addressed the optimization problem of cost, inventory, and schedules.
This synergy resulted in the establishment of an efficient, globally optimized supply chain system that can operate on a standard laptop in just 4 to 10 minutes—a remarkably quick turnaround for such a complicated task.
This innovative system achieved a 30% reduction in transportation costs for Toyota, highlighting the effectiveness of merging ML with OR in real-world applications. This hybrid approach allowed Toyota to lower operational expenses without the need for significant computational resources, a common barrier to implementing advanced solutions.
6.0. The Future of Hybrid AI: Small Models, Big Impact
As AI models grow larger, hybrid AI offers a refreshing alternative. Focusing on smaller, more efficient models, hybrid AI provides better performance while also promoting sustainability by using fewer computational resources.
As we look to the future, the hybrid AI approach may play a crucial role in advancing toward Artificial General Intelligence (AGI). By harnessing domain knowledge from operations research (OR), causal theory, and various mathematical disciplines, hybrid AI has the potential to address the existing limitations of machine learning (ML) and explore new horizons in intelligent systems.
7.0. Conclusion: Hybrid AI - The Key to Smarter, More Efficient Systems
The future of AI isn’t about scaling models to massive sizes; it’s about combining the best of multiple disciplines. Hybrid AI bridges the gap between data-driven learning and optimization, offering more robust, transparent, and resource-efficient solutions to real-world problems.
As AI continues to evolve, it’s important to look beyond the hype surrounding machine learning and recognize the untapped potential of hybrid AI. By focusing on smaller models and intelligent integrations, hybrid AI is setting the stage for a more sustainable and intelligent future.
8.0. Latest AI News
- 𝗔𝘂𝘁𝗼𝗻𝗼𝗺𝗼𝘂𝘀 𝗔𝗴𝗲𝗻𝘁𝘀: 𝗥𝗲𝘃𝗼𝗹𝘂𝘁𝗶𝗼𝗻𝗶𝘇𝗶𝗻𝗴 𝘁𝗵𝗲 𝗘𝗻𝘁𝗲𝗿𝗽𝗿𝗶𝘀𝗲 𝗟𝗮𝗻𝗱𝘀𝗰𝗮𝗽𝗲 𝗧𝗵𝗿𝗼𝘂𝗴𝗵 𝗦𝗲𝗹𝗳-𝗗𝗶𝗿𝗲𝗰𝘁𝗲𝗱 𝗘𝘅𝗽𝗹𝗼𝗿𝗮𝘁𝗶𝗼𝗻
- 𝗧𝗵𝗲 𝗔𝗜 𝗗𝗲𝗯𝗮𝘁𝗲 𝗼𝗳 𝗢𝘂𝗿 𝗧𝗶𝗺𝗲: 𝗖𝗼𝗺𝗽𝗹𝗶𝗮𝗻𝘁 𝗔𝘀𝘀𝗶𝘀𝘁𝗮𝗻𝘁𝘀 𝘃𝘀. 𝗦𝗰𝗶𝗲𝗻𝘁𝗶𝗳𝗶𝗰 𝗥𝗲𝘃𝗼𝗹𝘂𝘁𝗶𝗼𝗻𝗮𝗿𝗶𝗲𝘀
- Mistral AI has launched Mistral OCR, an Optical Character Recognition API that excels in understanding complex documents, including text, images, tables, and equations. It processes images and PDFs, extracting content in an ordered interleaved text and images format.
share this